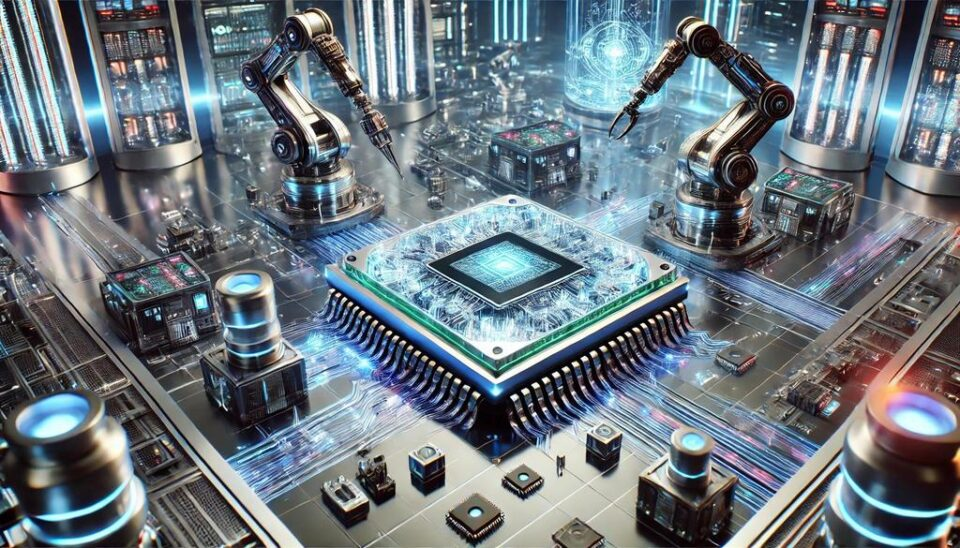
Nvidia has been the leading player in AI compute hardware with its GPUs. However, the Spring 2024 release of Cerebras Systems‘ third-generation chip, built on their advanced wafer-scale engine technology, is disrupting the market by providing enterprises with a new, competitive option.
This article examines the importance of Cerebras’ new product, comparing it to Nvidia’s solutions and those from Groq, another emerging AI hardware startup. It also highlights key factors enterprise decision-makers should consider when navigating this rapidly changing market.
The timing of Cerebras’ and Groq’s challenge is crucial. So far, most AI processing has focused on training large language models (LLMs), where Nvidia’s GPUs have dominated. However, in the next 18 months, the market is expected to shift as AI projects move from development to deployment. As AI workloads transition to inference, where speed and efficiency are key, the question arises: can Nvidia’s GPUs maintain their leadership?
Inference is the process where a trained AI model evaluates new data to generate results, such as responding in a chat with an LLM or guiding a self-driving car through traffic. Unlike training, which happens behind the scenes, inference drives real-time AI interactions and long-term decision-making. As the AI inference market is set for rapid expansion, experts predict it will reach $90.6 billion by 2030.
AI inference has traditionally relied on GPU chips, which excel at parallel computing, making them ideal for training large datasets. However, with the growing demand for intensive inference workloads, GPUs face challenges such as high power consumption, excessive heat generation, and costly maintenance.
Founded in 2016 by AI and chip design experts, Cerebras is a leader in AI inference hardware. Its flagship product, the Wafer-Scale Engine (WSE), is a groundbreaking AI processor that redefines inference performance and efficiency. The newly launched third-generation CS-3 chip, with 4 trillion transistors, is the largest neural network chip ever made—56 times bigger than the largest GPUs, resembling a dinner plate rather than a postage stamp. With 3000 times more on-chip memory, these chips can handle massive workloads independently, allowing for faster processing, better scalability, and lower power consumption.
The CS-3 chip shines when handling large language models (LLMs), reportedly processing an impressive 1,800 tokens per second for the Llama 3.1 8B model, significantly outperforming current GPU-based solutions. With prices starting at just 10 cents per million tokens, Cerebras is positioning itself as a strong competitor in the market.
The demand for speed
Given the high demand for AI inference, it’s no wonder that Cerebras’ remarkable statistics are capturing industry attention. The company has gained significant early traction, with its press kit highlighting praise from several industry leaders for its technology.
According to Kim Branson, SVP of AI/ML at GlaxoSmithKline, ‘Speed and scale change everything.’ The enhanced performance of Cerebras’ CS-3 has reportedly significantly improved the company’s capability to manage large datasets for drug discovery and analysis.
Denis Yarats, CTO of Perplexity, believes that ultra-fast inference is crucial for transforming search engines and user experiences. “Lower latencies lead to greater user engagement,” Yarats stated. “With Cerebras’ 20x speed advantage over traditional GPUs, we anticipate a fundamental shift in how users interact with search and intelligent answer engines.”
Russell d’Sa, CEO of LiveKit, emphasized that Cerebras’ ultra-fast inference has empowered his company to build advanced multimodal AI applications featuring voice and video interactions. “By pairing Cerebras’ top-tier computing power with LiveKit’s global edge network, we’ve created AI experiences with a more human touch, thanks to the system’s ultra-low latency.”
The competitive landscape: Nvidia, Groq, and Cerebras
Despite the strength of its technology, Cerebras operates in a highly competitive market. Nvidia’s dominance in AI hardware is well established, with its Hopper GPUs being essential for training and running AI models. Nvidia’s GPUs are offered through major cloud providers like Amazon Web Services, Google Cloud Platform, and Microsoft Azure, and its established market presence provides a substantial advantage in terms of ecosystem support and customer trust.
However, the AI hardware market is rapidly evolving and becoming more competitive. Groq, another emerging AI chip startup, is making an impact with its inference-focused language processing unit (LPU). Utilizing its proprietary Tensor Streaming Processor (TSP) technology, Groq offers strong performance benchmarks, energy efficiency, and competitive pricing.
Despite the impressive performance of Cerebras and Groq, many enterprise decision-makers may not yet be familiar with them, as they are newer to the market and still expanding their distribution channels, whereas Nvidia GPUs are widely available through major cloud providers. However, both Cerebras and Groq now offer robust cloud computing solutions and sell their hardware. Cerebras Cloud features flexible pricing models, including per-model and per-token options, enabling users to scale workloads without significant upfront costs. Similarly, Groq Cloud provides access to its advanced inference hardware via the cloud, claiming users can “switch from other providers like OpenAI by changing just three lines of code.” These cloud offerings allow decision-makers to explore cutting-edge AI inference technologies with lower costs and greater flexibility, making it easier to start despite their smaller market presence compared to Nvidia.
How do the choices measure up?
Nvidia
Performance: GPUs like the H100 are excellent at parallel processing tasks but fall short of the specialized speed offered by the CS-3 and LPU for AI inference.
Energy Efficiency: Although Nvidia has improved the energy efficiency of its GPUs, they are still more power-intensive compared to the offerings from Cerebras and Groq.
Scalability: GPUs offer high scalability, with established methods for linking multiple GPUs to handle large AI models.
Flexibility: Nvidia provides extensive customization options through its CUDA programming model and a broad software ecosystem, enabling developers to adapt GPU setups for a variety of computational tasks beyond just AI inference and training.
Cloud Compute Access: Nvidia GPU compute services are widely available through major cloud providers like Google Cloud Platform, Amazon Web Services, and Microsoft Azure.
Cerebras
Power: The CS-3 is an unprecedented powerhouse, featuring 900,000 AI-optimized cores and 4 trillion transistors. It can manage AI models with up to 24 trillion parameters and achieves peak AI performance of 125 petaflops, making it highly efficient for large-scale AI models.
Energy Efficiency: The CS-3’s large single-chip design minimizes inter-component traffic, significantly reducing energy consumption compared to GPU setups that rely on extensive networking.
Scalability: Cerebras’ WSE-3 is highly scalable, supporting clusters of up to 2,048 systems and delivering up to 256 exaflops of AI compute power.
Strategic Partnerships: Cerebras is partnering with major AI tools such as LangChain, Docker, and Weights and Biases, creating a strong ecosystem that facilitates rapid AI application development.
Cloud Compute Access: Currently available only through Cerebras Cloud, which offers flexible pricing options based on per-model or per-token usage.
Groq
Power: Groq’s Tensor Streaming Processor (TSP) is engineered for high-throughput AI inference with an emphasis on low latency. While it sets impressive benchmarks, it doesn’t match Cerebras in terms of token processing speeds.
Energy Efficiency: The TSP is optimized for energy efficiency, boasting up to 10 times greater efficiency compared to GPUs.
Scalability: Groq’s architecture supports scalability, allowing for the addition of more processors to enhance processing power.
Cloud Compute Access: Currently, Groq’s services are available exclusively through Groq Cloud.
Next Steps for Enterprise Decision-Makers
As the AI hardware landscape rapidly evolves, enterprise decision-makers should proactively assess their options. While Nvidia continues to lead the market, the rise of Cerebras and Groq presents compelling alternatives. Once considered the gold standard for AI compute, Nvidia GPUs now seem more like general-purpose tools rather than specialized ones. Purpose-built AI chips like the Cerebras CS-3 and Groq LPU could be indicative of the future direction.
Here are some steps business leaders can take to navigate the evolving AI hardware landscape:
Evaluate Your AI Workloads: Determine if your current and future AI workloads could benefit from the performance enhancements offered by Cerebras or Groq. If your organization heavily uses LLMs or requires real-time AI inference, these emerging technologies may provide substantial advantages.
Review Cloud and Hardware Options: Once you have a clear understanding of your workloads, assess the cloud and hardware solutions available from each vendor. Decide whether cloud-based compute services, on-premises hardware, or a hybrid approach best meets your needs.
Assess Vendor Ecosystems: Nvidia GPUs are widely available through cloud providers with a strong hardware and software ecosystem, while Cerebras and Groq are newer entrants in the market.
Remain Agile and Informed: Stay flexible in your decision-making and keep your team updated on the latest developments in AI hardware and cloud services.
The emergence of startup chip-makers Cerebras and Groq in the AI inference space marks a significant shift. Their specialized chips, such as the CS-3 and LPU, surpass the performance of Nvidia GPU processors, which have long been the industry standard. As AI inference technology evolves, enterprise decision-makers should regularly reassess their needs and strategies.